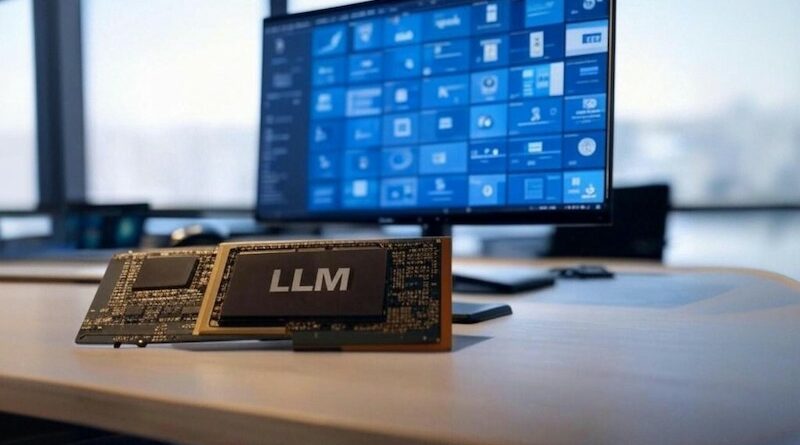
Generative AI and Innovation: From Large Language Models to Enterprise-Ready Platforms
Large Language Models (LLMs) are advanced generative AI systems capable of understanding, generating, and analyzing text with remarkable precision. Due to their unmatched power and versatility, LLMs are poised to revolutionize businesses and administrations in their daily operations. Still, to harness this promising technology in a secure and ROI-oriented manner, it is essential to implement a suite of functionalities that extend beyond the LLM itself. Understanding these steps can enlighten organizations in the “build or buy” dilemma.
The Mirage of Simplicity: An Underestimated Challenge
In the ever-evolving landscape of AI technologies, generative AI based on LLMs offers immense transformative potential for businesses and administrations. Essentially, this technology leverages all the unstructured information continuously produced: text documents or forms, graphics, tables, and more generally, any document representing the majority of information exchanged internally or with customers/users. Workflows involving such documents can be partially or totally automated thanks to GenAI, offering major productivity gains at every level of the organization.
The primary strength of generative AI tools, like ChatGPT, lies in their ability to conceal extreme technological sophistication behind a user-friendly chat interface. The availability of powerful open-source LLMs (such as Llama, Mistral, Qwen, Falcon) might suggest that building a complete internal GenAI solution is feasible.
However, transforming a raw LLM into an operational business application is a monumental challenge that should not be underestimated. A generic pre-trained model is merely a raw engine that requires a suite of technologies to address high-value business use cases in production.
Here, we will review 4 feature items that have to be built on top of LLMs in order to qualify as an enterprise-ready GenAI platform.
1/ Infrastructure: Critical Choices for Confidentiality and Scalability
While there is extensive literature on the initial training costs of LLMs that involve the continuous use of supercomputers equipped with GPU boards for several weeks, the infrastructure requirements for deploying LLMs in production are often overlooked despite being potentially significant. Indeed, once training is complete, using LLMs in production necessitates an appropriate GPU-based infrastructure that may become large when handling a large number of simultaneous queries. Commercial LLMs accessed through a pure Software-as-a-Service (SaaS) model eliminate direct infrastructure costs for the user but require data to be sent to the provider’s servers. For sensitive data, this approach may violate organizational data governance procedures, particularly in regulated sectors such as banking, insurance, healthcare, and public administration.
As an alternative, some GenAI providers offer their technology either on private cloud/single tenants or directly on-premise – within the client’s physical infrastructure – requiring an investment in servers equipped with GPUs. Properly choosing a future-proof infrastructure is crucial, as poor sizing can lead to either unacceptable latency or significant overcosts.
2/ Personalization : RAG Pipeline and Document Ingestion
Once installed, LLMs must be personalized with the company’s documentation. One of the most common applications of LLMs in businesses is the enhancement of document repositories through RAG (Retrieval Augmented Generation) systems. RAG combines generative AI with internal search engines, in order to create an intelligent dialogue system. Such modules enable users to simply ask questions related to the content of the documents, such as data extraction, summarization, document comparison, etc. While the basic principle of RAG systems is relatively simple, setting up an effective and scalable system for various document types (including those with specific layouts, tables, or graphs) is notoriously complex, involving multimedia document parsing and multiple calls to the LLM. It should be noted that using an LLM in RAG mode helps minimize the risk of “hallucinations” by displaying the sources employed to substantiate the answer.
More complex tasks, such as the automation of workflows, require the development of so-called “agents”, that involves the orchestration of various complementary tools, such as internet information search or the generation of self-correcting computer code. AI agents are currently an active field of research and are likely to become predominant in the future.
3/ Compliance : User Management, Data Access, and Regulatory aspects
Regulatory compliance is another fundamental aspect, particularly as AI regulations are being strengthened (GDPR, EU AI Act, etc.). Such regulations impose corporate liability and can result in extremely high fines for non-compliance.
In order to comply, tracing the origin of data at every stage, and understanding AI decisions are key requirements. Therefore, the auditability of systems has to be built-in. However, such transparency does not mean that all users have access to all data : Role-Based Access Control (RBAC) mechanisms must ensure that each user accesses only data that is relevant to the user’s organizational role.
4/ Maintenance and safety : How systems are monitored and maintained
Systems deployed in production must have advanced monitoring mechanisms that display usage by user category and diagnose component failures. Furthermore, built-in safety features must ensure that decisions based on AI are eventually reviewed and assessed by humans. Finally, given the extremely fast progress of generative AI technologies, AI platforms cannot be static. Maintaining and regularly updating systems without disrupting services and with appropriate documentation is essential.
Build or Buy: The Risks of an Internal Development Approach
Internally developing an AI platform may seem appealing for organizations seeking full control over their tools and budget, leveraging open-source LLMs and tools. This approach might be adequate when a large pool of highly technical engineers is available in-house, with skills ranging from GenAI, to software development and deployment (DevOps). However, while prototypes can be performed relatively easily, building a whole production-grade AI platform extends far beyond the core LLM, with large time scales and significant hurdles in the medium-to-long term.
As an alternative, working with external GenAI providers reduces operational risk and time-to-production. In a world where the adoption of generative AI has become a necessity, working with experts is not just a practical solution—it is an accelerator of digital transformation. Those who integrate this approach today will be the leaders of tomorrow.